Phenaki
A model for generating videos from text, with prompts that can change over time, and videos that can be as long as multiple minutes.
Read Paper
The water is magicalA photorealistic teddy bear is swimming in the ocean at San Francisco The teddy bear goes under water The teddy bear keeps swimming under the water with colorful fishes A panda bear is swimming under water |
Chilling on the beachA teddy bear diving in the ocean A teddy bear emerges from the water A teddy bear walks on the beach Camera zooms out to the teddy bear in the campfire by the beach |
Fireworks on the spacewalkSide view of an astronaut is walking through a puddle on mars The astronaut is dancing on mars The astronaut walks his dog on mars The astronaut and his dog watch fireworks |
Interactive example
Choose one combination of context words for creating a video about an astronaut.
All examples below use a model trained only on videos.
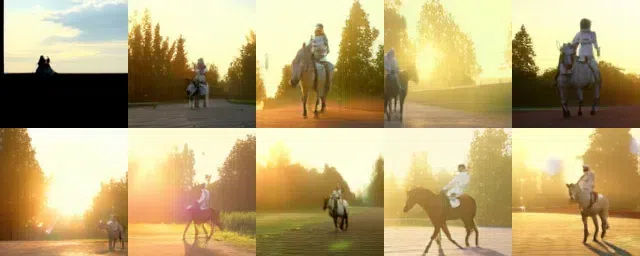
Generating video from a still image + a prompt
Input is the first frame, plus the prompt.
the eye of the cat |
the camera with the paw |
|
|
|
|
2 minute video
This 2-minute story was generated using a long sequence of prompts, on an older version of the model
![]() |
Lots of traffic in futuristic city. An alien spaceship arrives to the futuristic city. The camera gets inside the alien spaceship. The camera moves forward until showing an astronaut in the blue room. The astronaut is typing in the keyboard. The camera moves away from the astronaut. The astronaut leaves the keyboard and walks to the left. The astronaut leaves the keyboard and walks away. The camera moves beyond the astronaut and looks at the screen. The screen behind the astronaut displays fish swimming in the sea. Crash zoom into the blue fish. We follow the blue fish as it swims in the dark ocean. The camera points up to the sky through the water. The ocean and the coastline of a futuristic city. Crash zoom towards a futuristic skyscraper. The camera zooms into one of the many windows. We are in an office room with empty desks. A lion runs on top of the office desks. The camera zooms into the lion's face, inside the office. Zoom out to the lion wearing a dark suit in an office room. The lion wearing looks at the camera and smiles. The camera zooms out slowly to the skyscraper exterior. Timelapse of sunset in the modern city |
Abstract
We present Phenaki, a model capable of realistic video synthesis given a sequence of textual prompts. Generating videos from text is particularly challenging due to the computational cost, limited quantities of high quality text-video data and variable length of videos. To address these issues, we introduce a new causal model for learning video representation which compresses the video to a small representation of discrete tokens. This tokenizer uses causal attention in time, which allows it to work with variable-length videos. To generate video tokens from text we are using a bidirectional masked transformer conditioned on pre-computed text tokens. The generated video tokens are subsequently de-tokenized to create the actual video. To address data issues, we demonstrate how joint training on a large corpus of image-text pairs as well as a smaller number of video-text examples can result in generalization beyond what is available in the video datasets. Compared to the previous video generation methods, Phenaki can generate arbitrary long videos conditioned on a sequence of prompts (i.e. time variable text or a story) in open domain. To the best of our knowledge, this is the first time a paper studies generating videos from time variable prompts. In addition, the proposed video encoder-decoder outperforms all per-frame baselines currently used in the literature in terms of spatio-temporal quality and number of tokens per video.
Read the paper here.